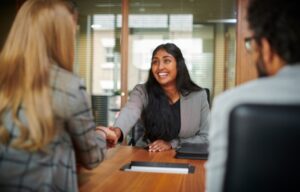
As technology advances, new job roles are created out of necessity. With companies and consumers continuing to explore and adopt artificial intelligence technology into their daily lives, the demand for advanced algorithms has skyrocketed. This is where machine learning engineers enter the equation.
Before we dive into what a machine learning engineer does, we need a brief overview of machine learning. According to Nvidia, machine learning is, “The practice of using algorithms to parse data, learn from it, and then make a determination or prediction about something in the world.” Essentially meaning, predictive models are fed data and then use that data to draw conclusions. For any machine learning algorithm to be useful it requires data and typically the more data used to train, the better the result. To delve deeper into machine learning, read this helpful article from our parent company, Quantilus.
Machine learning engineers are frequently thought of as a hybrid of data scientists and software engineers because they require skills from both roles, and commonly overlap responsibilities. Furthermore, with machine learning engineering being a newer role and dealing with cutting-edge technology, the position is continually evolving. The current state of the industry requires engineers very comfortable with trial and error. Most problems need to be approached from several different directions to find the optimal solutions and the solutions today, are not likely to be the solutions of tomorrow.
Machine learning engineers’ primary goal is to build and train a predictive model, by feeding the model data sets which are often created by data scientists. The more data provided to the model, the ‘smarter’ it becomes since it has more data to analyze and draw conclusions from. Simultaneous to providing the predictive model with data, the machine learning engineer will also examine the results the model creates to ensure its outputs are accurate and beneficial. Once the model reaches a certain level of readiness, the model can then be used commercially.
In summary, machine learning engineers are tasked with taking a prototype predictive model and training it until it provides knowledgeable, accurate, and beneficial conclusions, with little to no human interference. Also, it’s important to mention that a machine learning engineer does not always need to be a domain specialist, but does need to understand the models’ mathematics and desired outcome. For example, a maching learning engineer can build a model the identifies the intention of human speech with no linguistics or psychology background.
Additionally, specific skills help make a successful machine learning engineer. These skills are…
This is not an all-encompassing list but harnessing these skills will provide machine learning engineers with a solid foundation for the job. Also, note that a machine learning engineer’s responsibilities and skills will undoubtedly evolve as the technology advances.
Now that we know what a machine learning engineer is responsible for, let’s throw in an example for clarity and context. Netflix and Spotify are two companies that are renowned for their machine learning algorithms. Their algorithms recommend content to users based on their consumption history. For example, suppose a user regularly watches family-centric comedy shows that take place in the United Kingdom. In that case, Netflix will identify these various factors and offer show recommendations to the user based on this data. Machine learning engineers help create and train these algorithms to ensure they are producing accurate, tailored recommendations.
It is standard for machine learning engineers to possess a master’s degree at the minimum (Ph.Ds are also common), although higher education is not required for all machine learning roles. This is partly due to supply and demand. There is currently a high demand for machine learning engineers; however, the number of professionals with degrees and relevant experience is low. Therefore, some companies are willing to onboard individuals who might not have a college or grad-school degree(s) if they have the appropriate experience or can illuminate a solid understanding of the science.
If considering pursuing a degree, potential machine learning engineers might want to consider studying one of the following areas…
Machine learning engineering specific programs are also starting to crop up around the country, with schools such as Drexel University offering an MS in Machine Learning Engineering. Expect to see more universities offering similar programs in the coming years as the field continues to expand.
According to Indeed self-reported data, the average salary of a machine learning engineer in the United States is $150,000. However, in San Francisco, the average salary increases to nearly $200,000, and in New York City, the average salary is around $170,000.
Machine learning engineers can expect to see tremendous opportunity as the technology continues to gain adoption. From 2020-2024, the global machine learning industry is predicted to rise from $7.3 billion to $30.6 billion. That equates to a compound annual growth rate of 43%. Overall, the outlook for machine learning technology and related jobs is extremely healthy. With artificial intelligence and machine learning growing exponentially, the demand for machine learning engineers will continue to experience rapid growth.
If your company is looking to hire a machine learning engineer, or you are a machine learning engineer and looking for a career move, eNamix would love to talk with you. Contact us today at info@enamix.com to set-up a consultation with one of our senior account managers.
WEBINAR