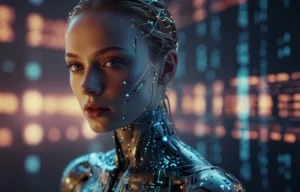
There’s no denying that OpenAI’s public release of ChatGPT in November 2022 opened the floodgates of generative AI powered by large language models (LLMs). Since then, the market has seen an exponential increase in the development of large language models. In fact, the LLM market is expected to grow from $6.4 billion in 2024 to $36.1 billion by 2030, according to a report from MarketstoMarkets™. However, within all this growth, a new trend is emerging: small language models (SLMs). These compact yet powerful AI models are leveling the playing field and making it more realistic for businesses to adopt generative AI into their workflows.
What are Small Language Models (SLMs)?
SLMs are compact versions of their larger counterparts, designed to provide similar functionality while requiring significantly fewer computational resources. These models, typically ranging from a few million to a few billion parameters are ideal for deploying in low-resource environments like local machines, mobile, and IoT devices. The training datasets needn’t be massive like large language models. SLMs can leverage limited datasets, particularly from specific domains, which can cut training time significantly.
All told, SLMs aim to balance performance and efficiency, making advanced natural language processing (NLP) capabilities accessible to a broader range of users and applications. Examples of SLMs include Phi-3 by Microsoft, Gemma by Google, Llama 3 by Meta, OpenELM by Apple, among several others. In an interview with TechRound about the Phi-3 SLMs, Sebastien Bubeck, Vice President of Generative AI Research at Microsoft, said, “These models are not only about size but about making powerful AI functionalities accessible at a lower cost and with greater ease.”
Why Choose an SLM over an LLM?
Small language models offer a range of compelling benefits that make them increasingly attractive for various applications. One of their primary advantages is resource efficiency. These models require significantly less computational power and memory compared to their larger counterparts, resulting in more cost-effective deployment and maintenance. This efficiency translates directly into lower cloud computing costs and enables these models to run on less powerful hardware, expanding their potential use cases.
Another key benefit is the speed of inference. Small LLMs, with their reduced number of parameters to process, can generate responses more rapidly. This quick turnaround is crucial for real-time applications such as chatbots or live text analysis, where immediate responses are essential for user engagement and satisfaction.
The ease of fine-tuning or training is another significant advantage. Smaller models can be adapted more quickly and with less data for specific tasks or domains. This flexibility allows businesses to efficiently tailor these models to their unique needs, enhancing their utility across various specialized applications. Furthermore, whereas LLMs may require massive and diverse datasets and months to train, SLMs leverage smaller, specific datasets that can train a model within weeks. Some other key benefits of SLMs include:
Who Are SLMs Best For?
There’s no hard and fast rule that says use an LLM versus an SLM. The truth is anyone and any sized business can leverage SLMs. The decision to go with an SLM will largely depend on your use case and circumstances. However, the mere existence of these models has made adoption of generative AI more accessible and affordable for all—not just the deep pocketed or highly-resourced organizations. That said, if you fall into one of the following groups, an SLM may be a viable choice for your needs.
What Tasks are Ideal for SLMs?
Small language models are particularly well-suited for a range of specific tasks that benefit from their efficiency and focused capabilities. These models excel in areas where quick processing, low resource usage, and specialized knowledge are more important than broad general knowledge.
The following tasks leverage the strengths of small LLMs—their speed, efficiency, and ability to specialize in specific domains. While they may not match the versatility of larger models for complex, open-ended tasks, they offer significant advantages in these focused applications, making them ideal for many business use cases where quick, efficient, and specialized language processing is required.
Key Takeaways
The rise of SLMs represents a significant shift in the AI landscape. These efficient, versatile models are democratizing access to advanced natural language processing capabilities, allowing businesses of all sizes to leverage the power of AI. By offering a balance of performance, efficiency, and accessibility, SLMs are enabling a new wave of innovative applications across various industries.
For organizations looking to implement AI solutions, SLMs offer an attractive entry point. They provide the opportunity to experiment with and deploy advanced NLP capabilities without the substantial investment required for larger models. As these technologies continue to evolve, they will undoubtedly play a crucial role in shaping the future of business.
WEBINAR