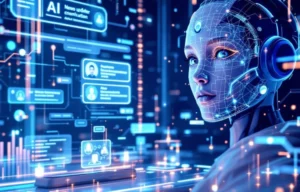
Data is produced at an unprecedented volume and tempo in today’s data-driven world. The Internet of Things (IoT) and the emergence of digital transformation have made data a valuable resource businesses can use to acquire understanding and spur expansion. The management and analysis of this data, particularly unstructured and semi-structured data, can be difficult. Here is where data lakes are useful.
A data lake is a centralized location where enormous amounts of unstructured, raw data are kept in their original form. It is a storage system that enables businesses to store and interpret data from various sources, like weblogs, sensors, social media, and consumer interactions, to name a few.
Â
Data lakes don’t require data to be pre-structured or pre-defined, in contrast to conventional data warehouses. Data is instead kept in its unprocessed state and can be altered and examined as required. As a result, data lakes provide enterprises with a flexible and scalable option for storing and processing huge volumes of data, facilitating the extraction of insights and value from their data assets.
Â
Data lakes are an excellent option for storing and processing massive volumes of data because they offer a few advantages. Here are some explanations for why your company could want a data lake:
A data lake absorbs, stores, and analyzes data from diverse sources. After being ingested, the data is kept in the data lake in its unprocessed state. Various methods, such as batch processing, real-time streaming, or direct data transmission, can add data to a data lake.
Â
A distributed file system, such as Hadoop Distributed File System (HDFS), Amazon S3, or Azure Data Lake Storage, frequently stores data in a data lake. These file systems offer a scalable and economical means of data storage, allowing businesses to store and handle massive volumes of data for less money.
Â
The data lake concept originated from the synergy between big data and Hadoop, an open-source platform for big data processing. Because the cloud offers performance, scalability, dependability, availability, a wide range of analytic engines, and significant economies of scale, Data Lakes are a task that is best implemented there. Higher security, quicker deployment times, higher availability, more regular feature/functionality updates, greater elasticity, greater geographic coverage, and pricing associated with actual usage are the main factors that customers cited as reasons why they saw the cloud as advantageous for Data Lakes.
The flexibility of the data lake architecture is offered in terms of data processing, analysis, and storage. The architecture enables a data-driven decision-making process by allowing data scientists, analysts, and business users to access the data lake to conduct research. It makes storing data from various sources simple because it enables companies to store raw data devoid of established patterns or schemas.
Data lakes are a modern and versatile architecture that consists of three layers – storage, processing, and access – where data is ingested, processed, and analyzed for insights. This architecture is highly scalable, fault-tolerant, and adaptable, making it suitable for companies of all sizes and industries. However, to successfully implement and manage a data lake, organizations must have a well-defined strategy, the right technology stack and follow best practices for data governance, security, and privacy.
Managing a data lake can be a complex task, requiring careful planning and implementation of best practices. To ensure efficient and effective data lake management, it is essential to establish a clear data governance policy, plan for scalability, select the appropriate storage option, and implement data quality controls. These best practices form the foundation for successfully managing a data lake and ensuring the reliability and accuracy of the data within it.
Implement data quality controls: A data lake relies heavily on data quality. Implement measures, such as data profiling, data validation, and data cleansing, to assure the consistency and accuracy of the data.
Â
In conclusion, a data lake may be necessary for enterprises for several reasons. Data lakes, in the first place, offer a scalable and adaptable method for handling and storing data. Traditional methods like data warehouses may no longer be appropriate for enterprises that need to store significant amounts of data in various formats due to the exponential growth of data. Second, data lakes give organizations a centralized location to store and retrieve data, making accessing and analyzing data from many sources simple. Third, data lakes offer a cost-effective method of storing data since they allow businesses to store data directly without using pricey ETL (extract, transform, and load) procedures. In general, a data lake is a crucial tool for businesses wishing to remain competitive in today’s data-driven world.
WEBINAR